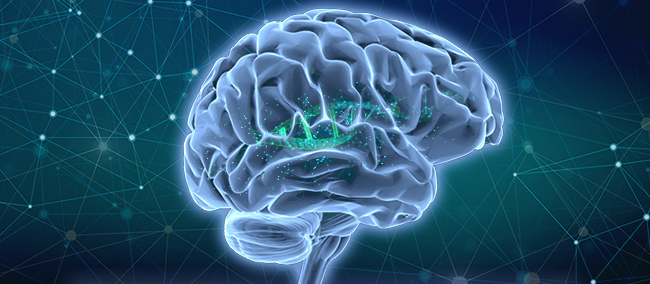
Schizophrenia is a complex and severe mental illness that affects approximately 1% of the global population. Often misdiagnosed for symptoms similar to other cognitive disorders, this condition lacks a valid biochemical laboratory test to support its clinical diagnosis.
Studies have shown that schizophrenia has both genetic and environmental components, suggesting that epigenetic dysregulation is likely an important contributor to its etiology. Thus, developing a diagnostic tool that can identify these abnormal epigenetic modifications could help detect the disease properly and earlier, allowing for therapeutic intervention that could reduce or delay the severity of the illness.
Unfortunately, one drawback to using epigenetic factors to test for diseases in the brain is that they are cell-type specific. Unlike genetic elements found in virtually every cell, epigenetic modifications can differ across various tissues. Therefore, testing blood samples may not provide accurate epigenetic information about the brain. This aspect presents a challenge for researchers looking to test tissues in inaccessible areas.
Newer research, however, has found that there are some regions in the genome that carry systemic interindividual epigenetic variations (called CoRSIVs), which are stable epigenetic polymorphisms set up during early development. Focusing on these regions, a group of scientists at Baylor College of Medicine (BCM) has developed an innovative strategy that uses machine learning to analyze blood for epigenetic variation, particularly DNA methylation, linked to schizophrenia. Their work is available to the public in the journal of Translational Psychiatry online.
“Because methylation patterns in CoRSIVs are the same in all the tissues of one individual,” said Dr. Robert A. Waterland, author and Professor of Pediatrics-Nutrition at BCM, “we can analyze them in a blood sample to infer epigenetic regulation on other parts of the body that are difficult to assess, such as the brain.”
DNA methylation is an important and widely studied epigenetic mechanism that affects gene expression by changing the profiles of proteins that attach to specific regions on the DNA. Considering that epigenetic factors can change in response to the environment, DNA methylation has been investigated to help explain the occurrence of schizophrenia – a disease that tends to run in families but is not linked to any one specific gene and may be partly inherited.
In a previous article, schizophrenia has been discussed in regards to why epigenetic factors must be considered a critical component of the disease. Plus, other papers we have reported on present research that explores DNA methylation in schizophrenia as well as other mental disorders.
“Our study is innovative in various ways,” explained Dr. Chathura J. Gunasekara, author and Bioinformatics Analyst at BCM. “We focused on CoRSIVs and also applied for the first time the SPLS-DA machine learning algorithm to analyze DNA methylation. As a scientist interested in applying machine learning to medicine, our findings are very exciting. They not only suggest the possibility of predicting risk of schizophrenia early in life, but also outline a new approach that may be applicable to other diseases.”
In their analysis, the researchers also factored into account methylation differences associated with smoking and antipsychotic drug use – something other studies had not considered.
By ruling out these potentially disconcerting factors, Waterman and his team established that the epigenetic patterns they identified at CoRSIVs in schizophrenia patients were most likely there before the disease was diagnosed. Indeed, this novel approach allows the researchers to identify epigenetic signals that correlate much stronger with schizophrenia than any other previous study.
“We consider our study a proof of principle that focusing on CoRSIVs makes epigenetic epidemiology possible,” said Waterland.
It’s hoped that the results from this study will lead to the development of a highly accurate blood test to prospectively identify individuals at risk for schizophrenia, or more generally, for a wide range of diseases.
Source: Chathura J. Gunasekara et al. (2021). A machine learning case–control classifier for schizophrenia based on DNA methylation in blood. Translational Psychiatry.
Reference: Homa Shalchi. A machine learning approach for predicting risk of schizophrenia using a blood test, Baylor College of Medicine. Aug 3, 2021.